Julia Set#
In the notebook I present different way to accelerate python code. This is a modified version from Loic Gouarin
The test case is the computation of the Julia set wikipedia
import os, sys
if sys.platform == 'darwin':
os.environ['CC'] = 'gcc-10'
os.environ['CXX'] = 'g++-10'
import warnings
warnings.filterwarnings('ignore')
import numpy as np
import matplotlib.pyplot as plt
import matplotlib.cm as cm
plt.rcParams['figure.figsize'] = (6,6)
Initialize Mesh#
nx, ny = 512, 512 # mesh
lim, maxit = 400, 2000 # limits
vmin, vmax = 0, 200
x = np.linspace(-1.6, 1.6, nx)
y = np.linspace(-1.6, 1.6, ny)
c = -0.772691322542185 + 0.124281466072787j
Pure Python#
def juliaset_python(x, y, c, lim, maxit):
"""
returns Julia set
"""
julia = np.zeros((x.size, y.size))
for i in range(x.size):
for j in range(y.size):
z = x[i] + 1j*y[j]
ite = 0
while abs(z) < lim and ite < maxit:
z = z**2 + c
ite += 1
julia[j, i] = ite
return julia
def plot_julia_set(julia):
plt.figure(figsize=(6,6))
plt.imshow(julia, cmap = cm.Greys, vmin=vmin, vmax=vmax)
plot_julia_set(juliaset_python(x, y, c, lim, maxit))
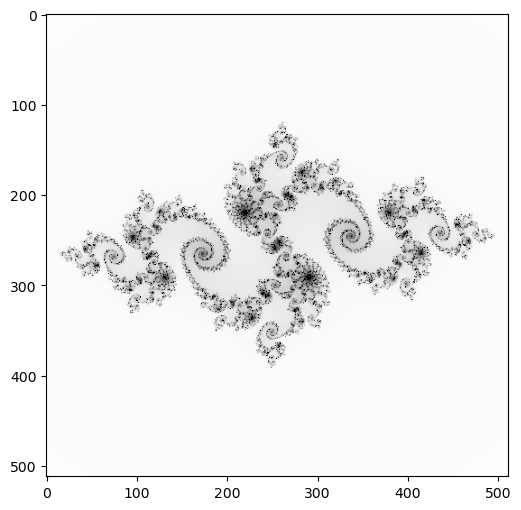
numba#
Numba will accelerate the pure python function just with
the decorator @jit
. Numba does everything for you.
from numba import jit
@jit(nopython=True, parallel=True)
def juliaset_numba(x, y, c, lim, maxit):
julia = np.zeros((x.size, y.size))
lim2 = lim*lim
c = complex(c) # needed for numba
for j in range(y.size):
for i in range(x.size):
z = complex(x[i], y[j])
ite = 0
while (z.real*z.real + z.imag*z.imag) < lim2 and ite < maxit:
z = z*z + c
ite += 1
julia[j, i] = ite
return julia
plot_julia_set(juliaset_numba(x, y, c, lim, maxit))
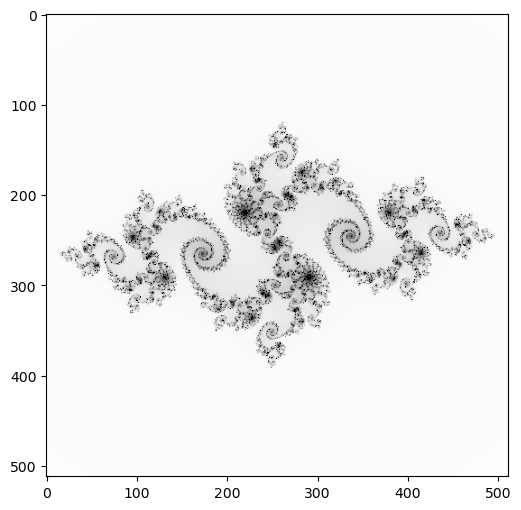
PyJulia#
PyJulia is a python module to import Julia function in your Python session. You can also run Julia code in a middle of a Jupyter notebook with a Python kernel.
To use pyjulia you need to install Julia and install PyCall.jl and REPL
julia> using Pkg
julia> ENV["PYTHON"] = "/usr/local/bin/python3.7"
julia> Pkg.add("PyCall")
julia> Pkg.add("REPL")
julia> Pkg.build("PyCall")
print the value of sys.executable
to know the python path. But the cell above could do the job.
import julia
julia.install()
from julia.api import Julia
jl = Julia(compiled_modules=False)
[ Info: Julia version info
Julia Version 1.10.4
Commit 48d4fd4843 (2024-06-04 10:41 UTC)
Build Info:
https://github.com/conda-forge/julia-feedstock
Note: This is an unofficial build, please report bugs to the project
responsible for this build and not to the Julia project unless you can
reproduce the issue using official builds available at https://julialang.org/downloads
Platform Info:
OS: Linux (x86_64-conda-linux-gnu)
Ubuntu 22.04.5 LTS
uname: Linux 6.5.0-1025-azure #26~22.04.1-Ubuntu SMP Thu Jul 11 22:33:04 UTC 2024 x86_64 x86_64
CPU: AMD EPYC 7763 64-Core Processor:
speed user nice sys idle irq
#1 3242 MHz 693 s 0 s 147 s 2956 s 0 s
#2 3128 MHz 535 s 0 s 149 s 3179 s 0 s
#3 2706 MHz 413 s 0 s 147 s 3186 s 0 s
#4 3210 MHz 408 s 0 s 134 s 3272 s 0 s
Memory: 15.606487274169922 GB (14380.125 MB free)
Uptime: 394.81 sec
Load Avg: 1.45 0.83 0.37
WORD_SIZE: 64
LIBM: libopenlibm
LLVM: libLLVM-15.0.7 (ORCJIT, znver3)
Threads: 1 default, 0 interactive, 1 GC (on 4 virtual cores)
Environment:
JULIA_CONDAPKG_BACKEND_BACKUP =
JULIA_CONDAPKG_BACKEND = System
JULIA_LOAD_PATH = @:@python-fortran:@stdlib
JULIA_LOAD_PATH_BACKUP =
JULIA_DEPOT_PATH_BACKUP =
JULIA_PROJECT_BACKUP =
JULIA_CONDAPKG_EXE = /usr/share/miniconda/bin/conda
JULIA_SSL_CA_ROOTS_PATH = /usr/share/miniconda/envs/python-fortran/ssl/cacert.pem
JULIA_DEPOT_PATH = /usr/share/miniconda/envs/python-fortran/share/julia:
LD_LIBRARY_PATH = /opt/hostedtoolcache/Python/3.9.20/x64/lib
JULIA_PROJECT = @python-fortran
JULIA_SSL_CA_ROOTS_PATH_BACKUP =
JULIA_CONDAPKG_EXE_BACKUP =
SELENIUM_JAR_PATH = /usr/share/java/selenium-server.jar
JAVA_HOME_11_X64 = /usr/lib/jvm/temurin-11-jdk-amd64
PKG_CONFIG_PATH = /opt/hostedtoolcache/Python/3.9.20/x64/lib/pkgconfig
GITHUB_PATH = /home/runner/work/_temp/_runner_file_commands/add_path_e4c41b58-e7b9-49f7-83d9-b89fce4f9a50
JAVA_HOME = /usr/lib/jvm/temurin-11-jdk-amd64
GRADLE_HOME = /usr/share/gradle-8.11.1
XDG_CONFIG_HOME = /home/runner/.config
ANT_HOME = /usr/share/ant
JAVA_HOME_8_X64 = /usr/lib/jvm/temurin-8-jdk-amd64
HOMEBREW_CLEANUP_PERIODIC_FULL_DAYS = 3650
DEPLOYMENT_BASEPATH = /opt/runner
ANDROID_NDK_LATEST_HOME = /usr/local/lib/android/sdk/ndk/27.2.12479018
HOME = /home/runner
CONDA_JL_HOME = /usr/share/miniconda/envs/python-fortran
JAVA_HOME_21_X64 = /usr/lib/jvm/temurin-21-jdk-amd64
GITHUB_EVENT_PATH = /home/runner/work/_temp/_github_workflow/event.json
JAVA_HOME_17_X64 = /usr/lib/jvm/temurin-17-jdk-amd64
ANDROID_NDK_HOME = /usr/local/lib/android/sdk/ndk/27.2.12479018
CONDA_JL_HOME_BACKUP =
HOMEBREW_NO_AUTO_UPDATE = 1
ANDROID_HOME = /usr/local/lib/android/sdk
PIPX_HOME = /opt/pipx
LEIN_HOME = /usr/local/lib/lein
PATH = /usr/share/miniconda/envs/python-fortran/bin:/usr/share/miniconda/condabin:/usr/share/miniconda/condabin:/opt/hostedtoolcache/Python/3.9.20/x64/bin:/opt/hostedtoolcache/Python/3.9.20/x64:/snap/bin:/home/runner/.local/bin:/opt/pipx_bin:/home/runner/.cargo/bin:/home/runner/.config/composer/vendor/bin:/usr/local/.ghcup/bin:/home/runner/.dotnet/tools:/usr/local/sbin:/usr/local/bin:/usr/sbin:/usr/bin:/sbin:/bin:/usr/games:/usr/local/games:/snap/bin:/home/runner/.dotnet/tools
SWIFT_PATH = /usr/share/swift/usr/bin
TERM = xterm-color
[ Info: Julia executable: /usr/share/miniconda/envs/python-fortran/bin/julia
[ Info: Trying to import PyCall...
┌ Error: `import PyCall` failed
│ exception =
│ ArgumentError: Package PyCall [438e738f-606a-5dbb-bf0a-cddfbfd45ab0] is required but does not seem to be installed:
│ - Run `Pkg.instantiate()` to install all recorded dependencies.
│
│ Stacktrace:
│ [1] _require(pkg::Base.PkgId, env::Nothing)
│ @ Base ./loading.jl:1926
│ [2] __require_prelocked(uuidkey::Base.PkgId, env::Nothing)
│ @ Base ./loading.jl:1812
│ [3] #invoke_in_world#3
│ @ ./essentials.jl:926 [inlined]
│ [4] invoke_in_world
│ @ ./essentials.jl:923 [inlined]
│ [5] _require_prelocked
│ @ ./loading.jl:1803 [inlined]
│ [6] _require_prelocked
│ @ ./loading.jl:1802 [inlined]
│ [7] macro expansion
│ @ ./lock.jl:267 [inlined]
│ [8] require(uuidkey::Base.PkgId)
│ @ Base ./loading.jl:1797
│ [9] top-level scope
│ @ /usr/share/miniconda/envs/python-fortran/lib/python3.9/site-packages/julia/install.jl:36
│ [10] include(mod::Module, _path::String)
│ @ Base ./Base.jl:495
│ [11] exec_options(opts::Base.JLOptions)
│ @ Base ./client.jl:318
│ [12] _start()
│ @ Base ./client.jl:552
└ @ Main /usr/share/miniconda/envs/python-fortran/lib/python3.9/site-packages/julia/install.jl:38
[ Info: Installing PyCall...
Resolving package versions...
Updating `/usr/share/miniconda/envs/python-fortran/share/julia/environments/python-fortran/Project.toml`
[438e738f] + PyCall v1.96.4
Updating `/usr/share/miniconda/envs/python-fortran/share/julia/environments/python-fortran/Manifest.toml`
[8f4d0f93] + Conda v1.10.2
[682c06a0] + JSON v0.21.4
[1914dd2f] + MacroTools v0.5.13
[69de0a69] + Parsers v2.8.1
[aea7be01] + PrecompileTools v1.2.1
[21216c6a] + Preferences v1.4.3
[438e738f] + PyCall v1.96.4
[81def892] + VersionParsing v1.3.0
[0dad84c5] + ArgTools v1.1.1
[56f22d72] + Artifacts
[2a0f44e3] + Base64
[ade2ca70] + Dates
[f43a241f] + Downloads v1.6.0
[7b1f6079] + FileWatching
[b27032c2] + LibCURL v0.6.4
[8f399da3] + Libdl
[37e2e46d] + LinearAlgebra
[d6f4376e] + Markdown
[a63ad114] + Mmap
[ca575930] + NetworkOptions v1.2.0
[de0858da] + Printf
[9a3f8284] + Random
[ea8e919c] + SHA v0.7.0
[9e88b42a] + Serialization
[fa267f1f] + TOML v1.0.3
[cf7118a7] + UUIDs
[4ec0a83e] + Unicode
[e66e0078] + CompilerSupportLibraries_jll v1.1.1+0
[deac9b47] + LibCURL_jll v8.4.0+0
[29816b5a] + LibSSH2_jll v1.11.0+1
[c8ffd9c3] + MbedTLS_jll v2.28.2+1
[14a3606d] + MozillaCACerts_jll v2023.1.10
[4536629a] + OpenBLAS_jll v0.3.23+4
[83775a58] + Zlib_jll v1.2.13+1
[8e850b90] + libblastrampoline_jll v5.8.0+1
[8e850ede] + nghttp2_jll v1.52.0+1
Precompiling project...
✓ CompilerSupportLibraries_jll
✓ Preferences
✓ VersionParsing
✓ PrecompileTools
✓ MacroTools
✓ Parsers
✓ JSON
✓ Conda
┌ Error: curl_multi_assign: 1
└ @ Downloads.Curl /usr/share/miniconda/envs/python-fortran/share/julia/stdlib/v1.10/Downloads/src/Curl/utils.jl:57
┌ Error: curl_multi_assign: 1
└ @ Downloads.Curl /usr/share/miniconda/envs/python-fortran/share/julia/stdlib/v1.10/Downloads/src/Curl/utils.jl:57
[2621] signal (6.-6): Aborted
in expression starting at /usr/share/miniconda/envs/python-fortran/lib/python3.9/site-packages/julia/install.jl:73
pthread_kill at /lib/x86_64-linux-gnu/libc.so.6 (unknown line)
raise at /lib/x86_64-linux-gnu/libc.so.6 (unknown line)
abort at /lib/x86_64-linux-gnu/libc.so.6 (unknown line)
uv__io_poll at /workspace/srcdir/libuv/src/unix/epoll.c:166
uv_run at /workspace/srcdir/libuv/src/unix/core.c:400
ijl_task_get_next at /usr/local/src/conda/julia-1.10.4/src/partr.c:478
poptask at ./task.jl:985
wait at ./task.jl:994
#wait#645 at ./condition.jl:130
wait at ./condition.jl:125 [inlined]
_wait at /home/conda/feedstock_root/build_artifacts/julia_1718579682237/work/usr/share/julia/stdlib/v1.10/FileWatching/src/FileWatching.jl:562
wait at /home/conda/feedstock_root/build_artifacts/julia_1718579682237/work/usr/share/julia/stdlib/v1.10/FileWatching/src/FileWatching.jl:590
#58 at /home/conda/feedstock_root/build_artifacts/julia_1718579682237/work/usr/share/julia/stdlib/v1.10/Downloads/src/Curl/Multi.jl:188
jfptr_YY.58_92826.1 at /usr/share/miniconda/envs/python-fortran/lib/julia/sys.so (unknown line)
jl_apply at /usr/local/src/conda/julia-1.10.4/src/julia.h:1982 [inlined]
start_task at /usr/local/src/conda/julia-1.10.4/src/task.c:1238
Allocations: 5502082 (Pool: 5496199; Big: 5883); GC: 8
---------------------------------------------------------------------------
PyCallInstallError Traceback (most recent call last)
Cell In[10], line 2
1 import julia
----> 2 julia.install()
3 from julia.api import Julia
4 jl = Julia(compiled_modules=False)
File /usr/share/miniconda/envs/python-fortran/lib/python3.9/site-packages/julia/tools.py:118, in install(julia, color, python, quiet)
116 return
117 elif returncode != 0:
--> 118 raise PyCallInstallError("Installing", output)
120 if not quiet:
121 print(file=sys.stderr)
PyCallInstallError: Installing PyCall failed.
** Important information from Julia may be printed before Python's Traceback **
Some useful information may also be stored in the build log file
`~/.julia/packages/PyCall/*/deps/build.log`.
%%file julia_set.jl
function escapetime(z, c, lim, maxit)
for n = 1:maxit
if abs(z) > lim
return n-1
end
z = z*z + c
end
return maxit
end
function juliaset_julia(x :: Vector{Float64}, y :: Vector{Float64},
c :: Complex, lim , maxit )
nx = length(x)
ny = length(y)
julia = zeros(Float64, (nx, ny))
Threads.@sync for i in eachindex(x)
Threads.@spawn for j in eachindex(y)
@inbounds z = x[i] + 1im * y[j]
@inbounds julia[j, i] = escapetime(z, c, lim, maxit)
end
end
return julia
end
Overwriting julia_set.jl
from julia import Main
juliaset_julia = Main.include("julia_set.jl")
Pythran#
Pythran is a Python-to-C++ translator
Add a comment line before your python function and it runs much faster.
Configuration#
~/.pythranrc
file on macos (gcc is installed with hombrew and pythran with pip)
[compiler]
include_dirs=/usr/local/opt/openblas/include
library_dirs=/usr/local/opt/openblas/lib
blas=openblas
CXX=g++-10
CC=gcc-10
%load_ext pythran.magic
%%pythran -fopenmp
import numpy as np
#pythran export juliaset_pythran(float64[], float64[],complex, int, int)
def juliaset_pythran(x, y, c, lim, maxit):
"""
returns Julia set
"""
juliap = np.zeros((x.size, y.size), dtype=np.int32)
#omp parallel for private(z, ite)
for j in range(y.size):
for i in range(x.size):
z = x[i] + 1j*y[j]
ite = 0
while abs(z) < lim and ite < maxit:
z = z**2 + c
ite += 1
juliap[j, i] = ite
return juliap
Fortran#
You need numpy and fortran-magic
%load_ext fortranmagic
On my mac i need to change compilers install with homebrew
Complex computation inside the loop are avoided on purpose. It takes time even with fortran.
%%fortran
subroutine juliaset_fortran(x, y, c, lim, maxit, julia)
real(8), intent(in) :: x(:)
real(8), intent(in) :: y(:)
complex(8), intent(in) :: c
real(8), intent(in) :: lim
integer, intent(in) :: maxit
integer, intent(out) :: julia(size(x),size(y))
real(8) :: zr, zi, limsq, cr, ci, tmp
integer :: ite, nx, ny
nx = size(x)
ny = size(y)
limsq = lim * lim
cr = real(c)
ci = imag(c)
do i = 1, nx
do j = 1, ny
zr = x(i)
zi = y(j)
ite = 0
do while (zr*zr+zi*zi < limsq .and. ite < maxit)
tmp = zr*zr - zi*zi
zi = 2*zr*zi + ci
zr = tmp + cr
ite = ite + 1
end do
julia(j, i) = ite
end do
end do
end subroutine juliaset_fortran
Fortran with OpenMP#
On Linux you don’t need to add -L/usr/local/lib
This is the same function from above with some openmp directives
%%fortran --f90flags "-fopenmp" --opt "-O3" --extra "-lgomp"
subroutine juliaset_fortran_omp(x, y, c, lim, maxit, julia)
real(8), intent(in) :: x(:)
real(8), intent(in) :: y(:)
complex(8), intent(in) :: c
real(8), intent(in) :: lim
integer, intent(in) :: maxit
integer, intent(out) :: julia(size(x),size(y))
real(8) :: zr, zi, limsq, cr, ci, tmp
integer :: ite, nx, ny
nx = size(x)
ny = size(y)
limsq = lim * lim
cr = real(c)
ci = imag(c)
!$OMP PARALLEL DEFAULT(NONE) &
!$OMP FIRSTPRIVATE(nx,ny,x,y,c,limsq,maxit,cr,ci) &
!$OMP PRIVATE(i,j,ite,zr,zi, tmp) &
!$OMP SHARED(julia)
!$OMP DO SCHEDULE(DYNAMIC)
do i = 1, nx
do j = 1, ny
zr = x(i)
zi = y(j)
ite = 0
do while (zr*zr+zi*zi < limsq .and. ite < maxit)
tmp = zr*zr - zi*zi
zi = 2*zr*zi + ci
zr = tmp + cr
ite = ite + 1
end do
julia(j, i) = ite
end do
end do
!$OMP END PARALLEL
end subroutine juliaset_fortran_omp
Numpy#
Vectorize version with numpy. You could get some overflow warnings
import itertools
def juliaset_numpy(x, y, c, lim, maxit):
julia = np.zeros((x.size, y.size), dtype=np.int32)
zx = x[np.newaxis, :]
zy = y[:, np.newaxis]
z = zx + zy*1j
for ite in itertools.count():
z = z**2 + c
mask = np.logical_not(julia) & (np.abs(z) >= lim)
julia[mask] = ite
if np.all(julia) or ite > maxit:
return julia
Cython#
Install Cython
Use %%cython -a
to highlight the Python-C and C-Python conversions.
Cython is not Python and not C, it is another language :-)
%load_ext cython
%%cython
import numpy as np
import cython
@cython.boundscheck(False)
@cython.wraparound(False)
def juliaset_cython(double [:] x, double [:] y, double complex c, double lim, int maxit):
cdef:
int [:, ::1] julia = np.zeros((x.size, y.size), dtype = np.int32)
double tmp, zr, zi, lim2 = lim*lim
double cr = c.real, ci = c.imag
int ite, i, j, nx=x.size, ny=y.size
for i in range(nx):
for j in range(ny):
zr = x[i]
zi = y[j]
ite = 0
while (zr*zr + zi*zi) < lim2 and ite < maxit:
zr, zi = zr*zr - zi*zi + cr, 2*zr*zi + ci
ite += 1
julia[j, i] = ite
return julia
As f2py we can use openmp with the Cython prange
function
%%cython -f -c-fopenmp --link-args=-fopenmp
import numpy as np
import cython
from cython.parallel import prange
from libc.stdlib cimport malloc, free
@cython.boundscheck(False)
@cython.wraparound(False)
def juliaset_cython_omp(double [:] x, double [:] y, double complex c, double lim, int maxit):
cdef:
int [:, ::1] julia = np.zeros((x.size, y.size), dtype = np.int32)
double tmp, zr, zi, lim2 = lim*lim
double cr = c.real, ci = c.imag
int i, j, nx=x.size, ny=y.size
int *ite
for j in prange(ny, nogil=True, schedule='dynamic'):
ite = <int *> malloc(sizeof(int))
for i in range(nx):
zr = x[i]
zi = y[j]
ite[0] = 0
while (zr*zr + zi*zi) < lim2 and ite[0] < maxit:
zr, zi = zr*zr - zi*zi + cr, 2*zr*zi + ci
ite[0] += 1
julia[j, i] = ite[0]
free(ite)
return julia
Set number of threads used for parallel functions#
%env OMP_NUM_THREADS=4
%env JULIA_NUM_THREADS=4
env: OMP_NUM_THREADS=4
env: JULIA_NUM_THREADS=4
import pandas as pd
from collections import defaultdict
from tqdm import tqdm_notebook as tqdm
results = defaultdict(list)
nx, ny = 1024, 1024 # increase mesh size
x = np.linspace(-1.6, 1.6, nx)
y = np.linspace(-1.6, 1.6, ny)
functions = [juliaset_numpy,
juliaset_fortran,
juliaset_fortran_omp,
juliaset_cython,
juliaset_cython_omp,
juliaset_numba,
juliaset_pythran,
juliaset_julia]
for f in tqdm(functions):
_ = %timeit -q -n 1 -o f(x, y, c, lim, maxit)
results['etime'] += [_.best]
results = pd.DataFrame(results, index=list(map(lambda f:f.__name__[9:],functions)))
results["speed_up"] = [results.etime["numpy"]/t for t in results.etime]
results.sort_values(by="speed_up",axis=0)
etime | speed_up | |
---|---|---|
numpy | 3.744096 | 1.000000 |
pythran | 0.059130 | 63.320112 |
numba | 0.056067 | 66.778523 |
fortran | 0.052708 | 71.035016 |
cython | 0.052008 | 71.990972 |
julia | 0.025038 | 149.536701 |
cython_omp | 0.009314 | 401.970603 |
fortran_omp | 0.007442 | 503.092445 |